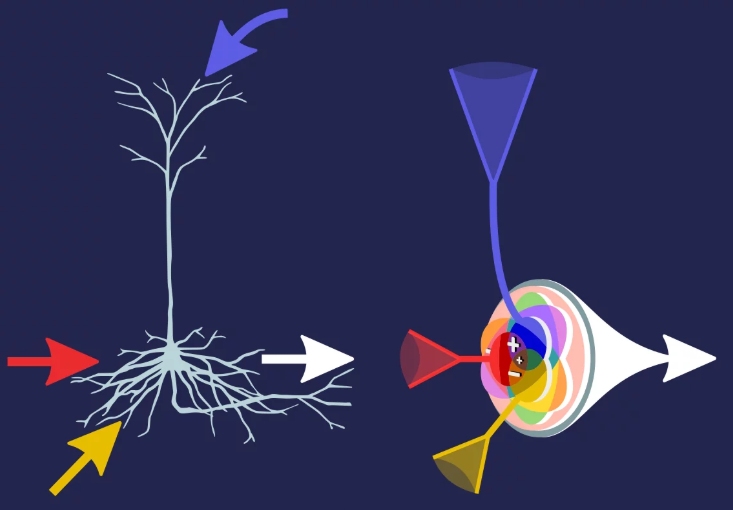
Imagine if artificial neurons could learn like the ones in our brains, adapting, making decisions on their own, and figuring things out without needing a central command. That’s exactly what scientists are working on with a new kind of artificial neuron called infomorphic neurons. Unlike traditional artificial neural networks, these neurons don’t need an external system to guide their learning. Instead, they learn in a self-organized way, just like biological neurons do.
How Do Infomorphic Neurons Learn?
Think about how a group of friends might teach each other a new game. Instead of one person acting as the instructor, everyone picks up the rules by observing, trying things out and adjusting based on what works. That’s kind of how infomorphic neurons operate. Each neuron learns independently by analyzing signals from its immediate neighbors in the network. There’s no one controlling or telling them what to do, rather, they just figure it out as they go.
Here’s how they are working:
- They make independent decisions. Each neuron decides for itself which inputs matter and which don’t. No one is spoon-feeding it information.
- They learn from their surroundings. Like real neurons in the brain, these artificial neurons pick up patterns from their neighboring connections.
- They follow simple learning goals. Scientists set broad objectives, but the neurons come up with their own rules to achieve those goals.
- They adjust based on information theory. A special measure helps neurons determine whether to collaborate with neighbors, specialize in a specific task or reinforce existing patterns.
- They self-organize. Over time, the neurons specialize and work together to help the whole network function efficiently.
This way of working is like how brain cells, especially certain types called pyramidal cells, learn from the connections they make with each other instead of depending on a central authority.
Infomorphic Neurons: Unlocking Real-Time Learning in AI
One big reason biological neurons outperform artificial ones is their ability to adapt in real-time. They don’t wait for a central system to update their learning rules. Instead, they adjust on the fly based on what’s happening around them. This kind of localized learning makes them more efficient and resilient when dealing with new or unexpected situations.
Artificial neural networks, on the other hand, usually require an external trainer to fine-tune their learning. If you want to improve an AI model, you typically have to feed it tons of labeled data and tweak its parameters manually. Infomorphic neurons offer a different approach by allowing each neuron to figure things out on its own, making the system more adaptable and efficient.
Self-Learning AI Inspired by Biology
Instead of being micromanaged, these neurons work with simple, broad learning objectives. Scientists don’t give them step-by-step instructions, they just provide a general framework, and the neurons do the rest. Here’s what they focus on:
- Redundancy: Some neurons reinforce patterns by sharing the same information with their neighbors.
- Collaboration: Others work together in a coordinated way to process complex information.
- Specialization: Some focus on specific details, handling unique pieces of information within the network.
By balancing these three behaviors, the network as a whole can learn efficiently without needing constant human intervention.
Infomorphic Neurons: Advancing Both AI and Neuroscience
Beyond improving artificial intelligence, infomorphic neurons help us understand how real neurons learn. One of the biggest challenges in neuroscience is figuring out how learning actually happens in the brain. By designing artificial systems that learn in a similar way, scientists can test theories about biological learning and refine their models.
At the same time, this could lead to more efficient AI. Today’s artificial neural networks consume a lot of power. Training deep learning models can require energy equivalent to hundreds of thousands of human brains. If we can teach AI to learn more like our brain cells do it could lead to smarter systems that use less energy.
Takeaway
Infomorphic neurons are a step toward making artificial learning more flexible, independent, and efficient. Instead of relying on a central trainer, these neurons learn from each other, just like real neurons do in the brain. They follow broad learning goals but find their own way to achieve them. And by doing so, they offer insights into both AI and neuroscience.
We’re still in the early stages, but this research could change how we think about machine learning. If AI can become more self-sufficient and adaptive, who knows what new possibilities might emerge? Or what if machines learn the way we do, will they start to think in ways we never expected?
Source: Max Planck Institute for Dynamics and Self-Organization