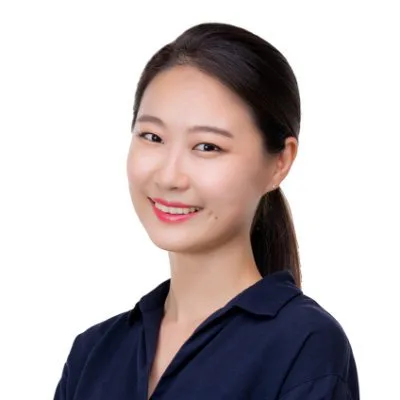
I’m excited to introduce our latest interviewee, Dr. Eunhye Baek, whose impressive academic journey and cutting-edge research make her a standout in the field of electrical engineering and nanotechnology. Dr. Baek studied Electrical Engineering at Pohang University of Science and Technology (POSTECH) from 2007 to 2010. In January 2011, she joined Professor Yoon-Ha Jeong’s group at POSTECH, where she focused on the biological functionalization of silicon nanowire BioFETs to detect biomolecules with high sensitivity.
In March 2012, Dr. Baek expanded her research horizons by collaborating with the InnovaSens group led by Professor Gianaurelio Cuniberti at TU Dresden, working on photoswitchable nano-devices. By February 2013, she had earned her master’s degree with a thesis on the “Optoelectronic Switching of Porphyrin-Coated Silicon Nanowire Field Effect Transistors.” Not one to rest on her laurels, Dr. Baek joined the Chair of Materials Science and Nanoelectronics as a Ph.D. student in March 2013, where she is developing innovative silicon nanowire-based hybrid devices decorated with functional organic materials, including photochromic molecules.
In 2019, she joined the Center for Brain-inspired Computing Research at Tsinghua University for her postdoctoral research. She is now continuing her work on neuromorphic network intelligence at the Center for Complex Network Intelligence, Tsinghua Brain and Intelligence Laboratory.
Her recent work on Brain-like Artificial System: Dendristor to Mimic Brain’s Dendritic Computations caught our attention. Despite her hectic schedule, she graciously agreed to an interview. Please find our insightful conversation below.
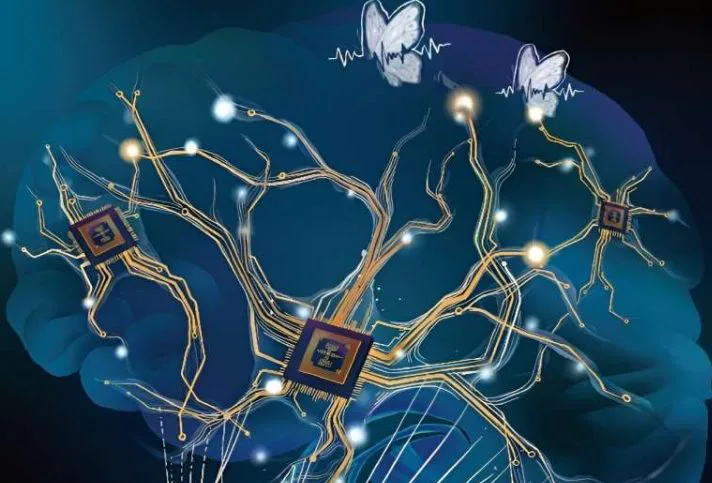
What initially sparked your interest in brain-inspired neuromorphic intelligent systems, and what motivated you to pursue it as a career?
When I was in a high school, I read a Korean sci-fi comic where the main character discussed with a biological AI system called “the Old Library”, built with the memories of people. This inspired me greatly, as I had many questions about the world and dreamed of creating a hardware system that could think like human being but possess the vast information of the universe.
When I went to graduate school, there weren’t many labs studying the neuromorphic field in electronics and electrical engineering, so I joined a biosensor group that could combine biological system with electronics. I thought this would give me a chance to develop a system similar to a brain.
Initially, I tried to develop a biosensor with a cell-like environment. I studied materials where biological reactions could occur and combined them with a transistor device. Interestingly, due to the mobile ions doped in the materials, the device showed plasticity similar to that in a neuron cell. It felt like destiny, because I didn’t do it on purpose, but I jumped into the neuromorphic field by chance, which had been my long-term dream.
The more I study the brain to develop a brain-like neuromorphic system, the more insights I gain about ourselves. This might be the key difference between developing AI and brain-like systems. In other words, the gap between AI and the biological brain is still significant. Until “The Old Library” is established, I will continue to pursue understanding and developing a neuromorphic intelligent system.
How do neuromorphic systems draw inspiration from the human brain, and what are the key principles they mimic?
There are several aspects how neuromorphic systems mimic the human brain based on scale. One aspect is the top-down approach, in which researchers try to mimic the brain’s holistic learning operations, particularly in the prefrontal cortex. They mimic the connectivity of the neurons and the parallel processing of the brain, which inspires the development of artificial neural networks. In this approach, a neuron is modeled as a point neuron with a mathematical activation function. The focus is on learning and classifying static or dynamic patterns. Researchers attempt to scale up their systems on integrated circuits to process massive amount of data. In-memory computing chips or neuromorphic accelerators fall into this field. It can process massive amounts of data, but the biological details are quite far from those of the biological brain.
The other aspect is the bottom-up approach, where researchers try to mimic operations and structure of building blocks of the brain, such as synapses and neurons. Here, the material properties of the artificial synapse or neuron become important, as their operation depends on the physics of materials. The artificial devices mimic synaptic or neuronal plasticity in a more biologically plausible way. In this approach, an artificial neuron has more complex biological functions, such as nonlinear integration, intrinsic plasticity, or spike generations. This approach can be closer to the biological system, but scaling up remains a challenge.
Our recent study published in Nat. Electron. (2024) follows this bottom-up approach. We mimic neuron’s functionality, including dendritic computation. This deviates from the mathematical point-neuron model by incorporating dendritic morphological properties, such as branch-specific plasticity or signal mapping through multiple dendritic branches. Thanks to these dendritic properties, our neuromorphic system can compute the direction of a moving object signal with a comparably small number of neurons. This highlights the advantage of using more brain-like system.
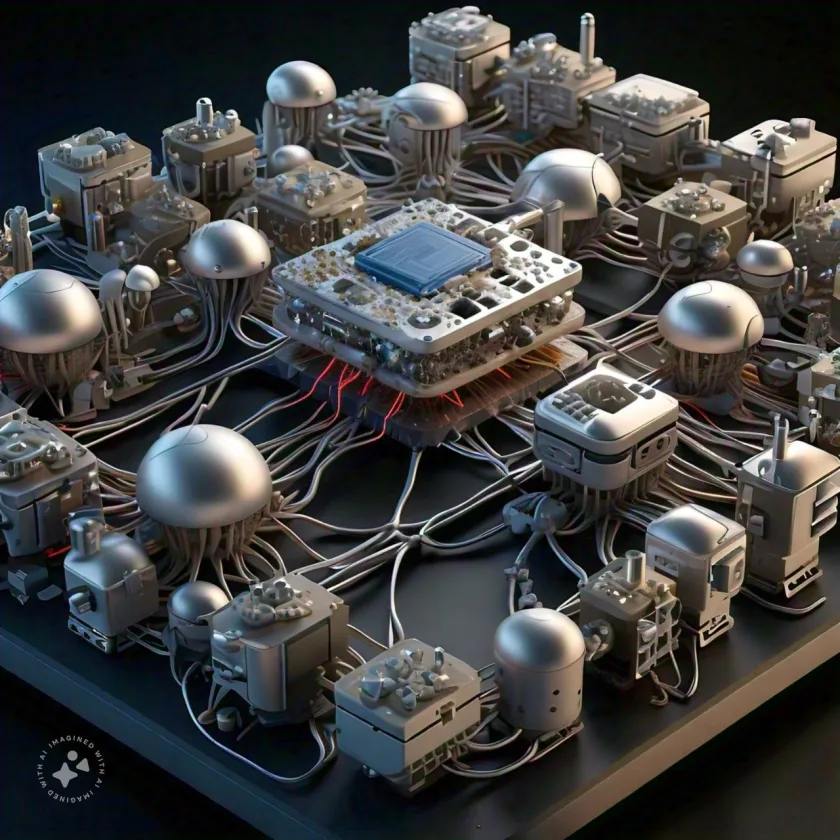
How do you plan to integrate silent synapses in a way that mimics their natural function?
We designed the silent synapse as an input switch that activates when the dendritic branch potential reaches a specific threshold. In our system, the dendritic branch potential is linked to the effective gate voltage on the transistor device, influenced by the sol-gel film’s potential on the transistor. Currently, this is implemented with a circuit model, but we believe it can be realized with a memristor, which becomes highly conductive when a specific voltage threshold is reached.
How does your neurotransistor emulate the intrinsic plasticity of the neuronal membrane? Ref: Intrinsic plasticity of silicon nanowire neurotransistors for dynamic memory and learning functions
Our neurotransistor is built with a silicon nanowire transistor device covered by ion-doped sol-gel film used as the gate dielectric material. The transistor’s conductivity (output current) is thus modulated by changes in electric potential within the film. The gate voltage applied to the sol-gel film induces the ion polarization within the film, and this polarization is thermally maintained for a period of time. We guess that the ions are temporarily trapped in the amorphous sol-gel film, providing a short-term memory effect. Due to these material properties, the neurotransistor’s conductivity dynamically changes, following a sigmoidal trajectory over time with pulse inputs. This behavior is similar to neuronal intrinsic plasticity, where the firing rate changes in a sigmoidal manner in response to input signals.
What are the key advantages of using your neurotransistor over other artificial synapses in neuromorphic systems?
The neurotransistor mimics neuronal dynamic behavior, distinguishing it from artificial synapses. While artificial synapses have been highlighted as a key element for learning, we have demonstrated that artificial neurons are another important component due to their intrinsic plasticity. Using the internal state change of neurotransistors, we have shown that their internal dynamics can be altered through learning, which can be utilized for pattern classification (Nat.Electron.2020). By extending the sol-gel film structure of the neurotansistor, we developed the Dendristor model, which performs dendritic computation (Nat.Electron. 2024). The dendritic neuron and neural circuit model incorporate more comprehensive neuronal operations and morphological characteristics.
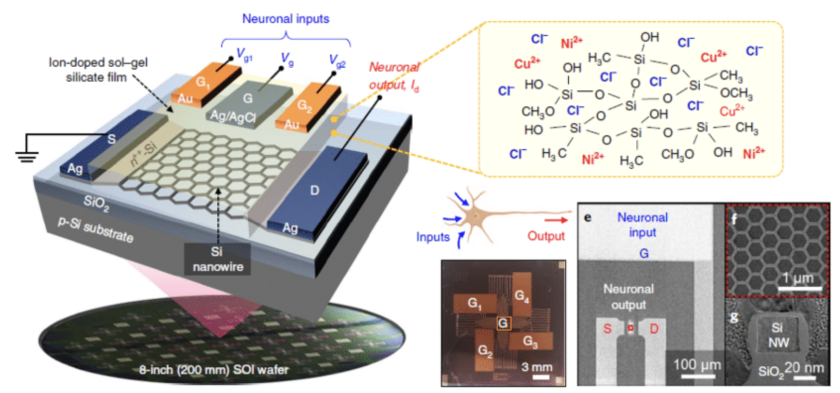
What are your other interests besides academic commitments… reading, painting, gardening, skiing maybe?
I love drinking Chinese tea. The world of Chinese tea is incredibly deep and rich. It always astonishes me with its variety, sensitivity and delicate tastes. Depending on processing methods such as withering, pan-firing, rolling, oxidation and drying, Chinese tea can exhibit tremendous range of flavors and fragrances. My current favorite is green tea. Even within green tea, the shapes and tastes are so diverse depending on their production area. Tea helps me relax and work, embracing and comforting me every time I drink it.
Quick bits 🙂
What would you do on Mars for fun?
Work on terraforming projects.
What books should I read in 2024?
Books on linear algebra as it is fundamental for most of future technology.
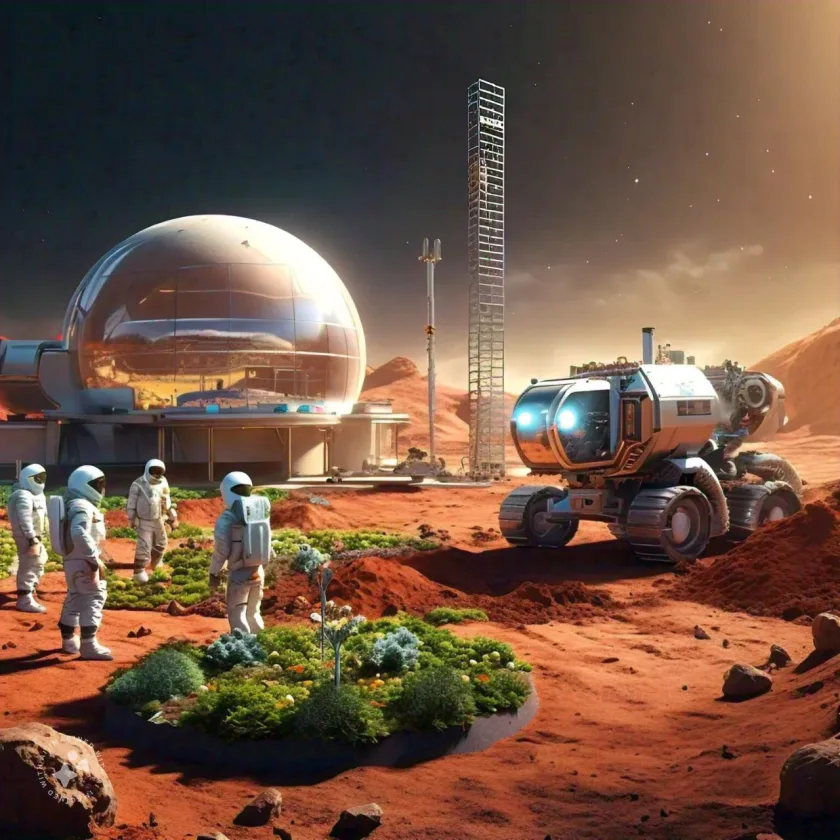
(Wow! Thank you, Dr. Baek, for an incredibly inspiring conversation! Your work is a true source of inspiration. We eagerly anticipate our next visit to witness more of your innovative research. Until then, we extend our best wishes for your continued success in all your future endeavors.)