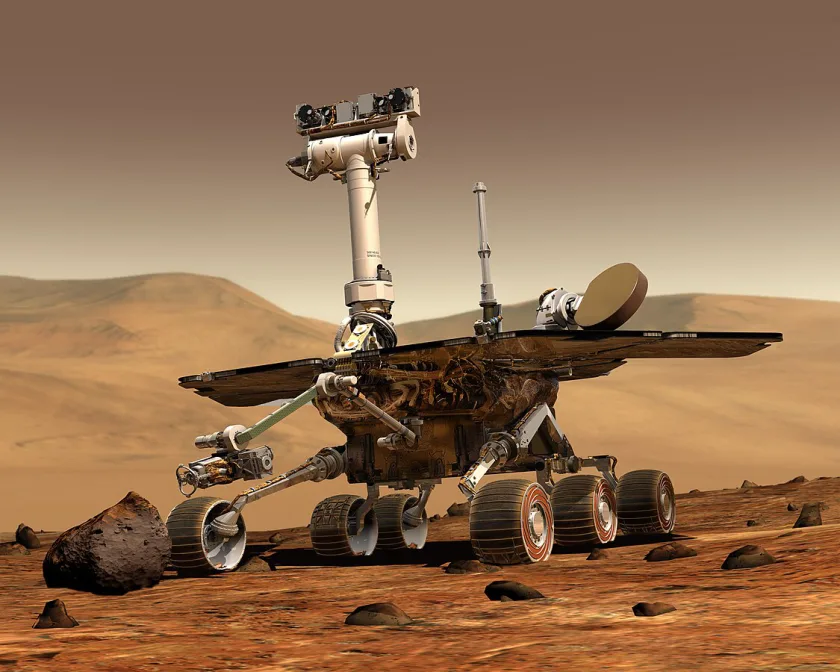
Rovers on Mars are constantly monitored and maneuvered by humans on Earth. However, robots on missions to Saturn or Jupiter’s moons can’t get timely commands from Earth.
What if these machines could make decisions on the fly? Instead of waiting for an “order”, how about these bots making autonomous choice?
Thinking on these lines of thought, researchers at the University of Illinois Urbana-Champaign came up with a cool way for these robots to decide where and how to collect terrain samples on their own.
Instead of trying to simulate every possible scooping scenario, the researchers taught the robots to quickly learn how to scoop on unfamiliar materials they encounter.
In addition to adapting to changing landscapes, these machines also learn about the properties of the materials they came across.
Using this innovative learning approach, the robots can quickly grasp the technique of scooping a new material with minimal attempts.
When they make errors, they learn from them, understanding that certain areas should not be scooped, prompting them to explore alternative locations.
Deep Gaussian Process Model for Real-Time Decision-Making
The researchers developed a deep Gaussian process model, which is trained using an offline database through deep meta-learning with controlled deployment gaps.
In the proposed method, the training set is divided into mean-training and kernel-training subsets multiple times.
The learning process focuses on adjusting the kernel parameters to minimize the differences between the actual data and the predictions made by the mean models.
When deployed, the decision-maker employs the trained model and adjusts it based on the new data acquired online. This adaptation ensures the model’s effectiveness in real-time decision-making.
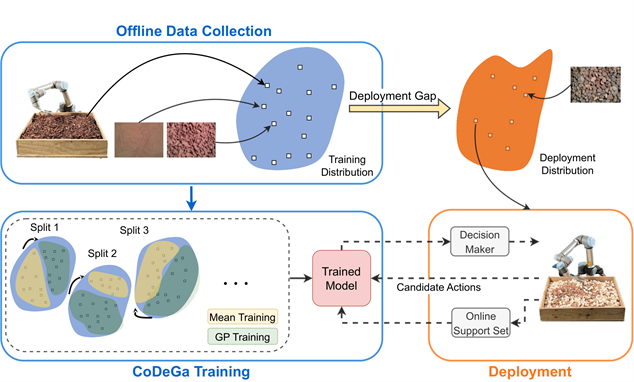
Limited Knowledge of Ocean Worlds like Europa
The lack of knowledge about ocean worlds like Europa poses a significant challenge for this research. The resolution of the best available image of Europa is around 256 to 340 meters per pixel, which is insufficient for accurately identifying terrain features.
Thus, scientists have limited information about the specific characteristics and composition of these extraterrestrial environments.
This limited understanding makes it difficult to accurately predict and adapt to the unique conditions and challenges that may be encountered during robotic exploration missions.
Melkior Ornik, on the researcher, stated that while Europa’s surface is known to be composed of ice, the specific nature of the ice, whether it consists of large blocks or fine particles like snow, remains uncertain. Furthermore, the composition beneath the ice layer is also unknown.
Robotic Learning through Adaptability and Strategic Shifting
As part of their experimentation, the research team employed a method where they concealed various materials beneath a layer of a different substance.
The robot, limited to observing only the top layer, made an assumption that it was suitable for scooping and proceeded accordingly.
However, upon actually executing the scoop and encountering the bottom layer, the robot learned from the experience that the material was unsuitable for scooping.
As a result, it adapted its strategy by shifting to a different area, demonstrating the learning and adaptive capabilities of the robot, as described by Thangeda, a Ph.D. student in the Department of Aerospace Engineering.
NASA’s Battery-Powered Rovers for Europa
To minimize the risk of contamination of the ocean worlds, NASA plans to send battery-powered rovers instead of nuclear-powered ones to Europa.
The limited lifespan of batteries (around 20 days) compared to nuclear power supplies is a crucial factor in decision-making.
Moreover, continuous communication between Earth and the rover is time-consuming. Therefore, making the robot’s autonomy even more essential.
The learning-to-learn approach employed in this research is remarkable as it enables the robot to achieve excellent scooping performance on unfamiliar terrains with minimal online experience. Thus, surpassing non-adaptive methods and other state-of-the-art meta-learning techniques.
A database consisting of 6,700 points was created, including 12 unique materials and terrains composed of one or more materials.
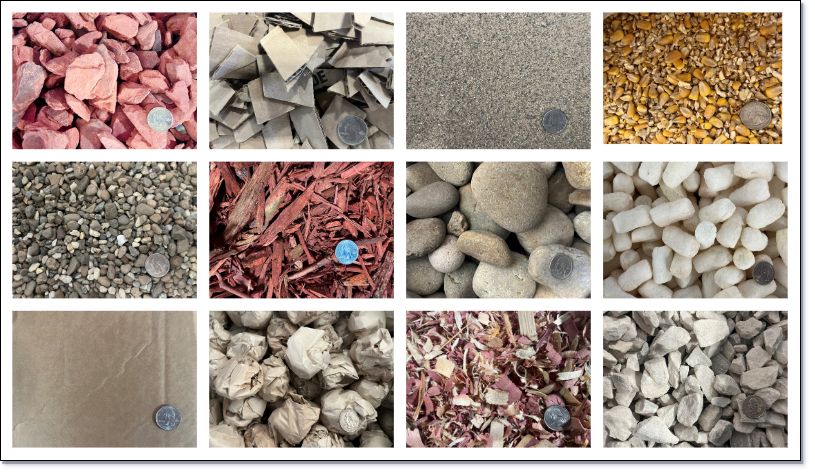
Generate Extensive Simulation Database
At the University of Illinois, researchers utilized a robot designed like a lander’s arm, equipped with sensors from the Department of Computer Science.
This robot performed scooping experiments on a wide range of materials, including grains of sand as small as 1 millimeter and rocks as large as 8 centimeters.
It also tested materials of different volumes, such as shredded cardboard and packing peanuts.
As a result, they generated a simulation database comprising 6,700 data points, with 100 points for each of the 67 unique terrains.
First Open-Source Dataset on Granular Media
According to Thangeda, the research team is proud to be the first to open-source a large-scale dataset on granular media. This way, it’ll be easily accessible for others to utilize in their applications.
The model developed by the team will be deployed and tested at NASA’s Jet Propulsion Laboratory’s Ocean World Lander Autonomy Testbed.
Ornik expressed their interest in advancing autonomous robotic capabilities on extraterrestrial surfaces, particularly on challenging terrains. This unique method holds great potential in supporting NASA’s exploration of ocean worlds.
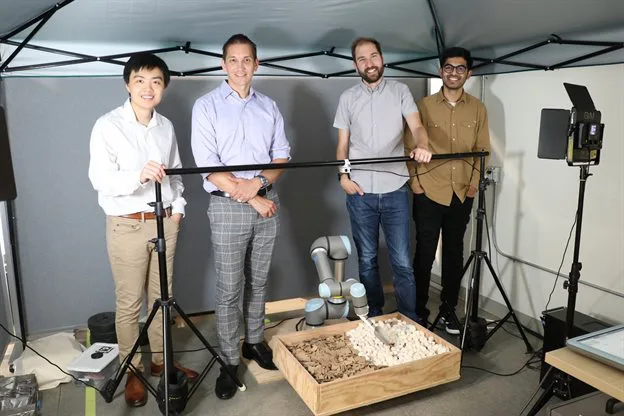
Takeaway
This research holds great significance as it enables the transfer and adaptation of knowledge and methodologies from Earth to extraterrestrial bodies.
This becomes especially valuable due to the limited information available prior to the lander’s arrival and the short lifespan of the lander’s batteries. The ability to learn and make autonomous decisions becomes vital under such circumstances.
[…] Robots making Autonomous Decisions on Extraterrestrial Missions: Learning to Learn […]